Josef Ressel Center for Symbolic Regression
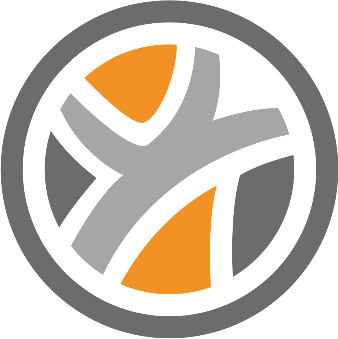
Project duration from 2018-01-01 to 2022-12-31
Funded by: CDG
Partners:
- Miba Frictec GmbH
- AVL List GmbH
- EREMA Engineering Recycling Maschinen u Anlagen GmbH
https://symreg.at
Within the Josef Ressel Centre for Symbolic Regression we plan to develop new symbolic regression algorithms as well as a methodological and technical framework for incremental model adaptation for handling concept drift. We will apply the newly developed algorithms and frameworks for modelling powertrains and friction systems.
Team
- Gabriel Kronberger
- Postdocs: Bogdan Burlacu, Michael Kommenda
- PhD students: Florian Bachinger, Christian Haider, David Jödicke, Lukas Kammerer
Christian Haider, Florian Bachinger, Lukas Kammerer, Michael Kommenda, Eva-Maria Holzleitner, Bogdan Burlacu, Gabriel Kronberger) (© Petra Wiesinger, FH OÖ)
Partners
The Josef Ressel Centre for Symbolic Regression is a cooperation of four companies. It is led by University of Applied Sciences Upper Austria, Hagenberg Campus (FH OÖ). The three company partners are AVL List GmbH, Miba Frictec GmbH, and EREMA Engineering Recycling Maschinen und Anlagen GmbH. They provide relevant business cases and expert knowledge as well as infrastructure (testing benches) and data. The company partners also provide 50% of the financial funding for the project. The other 50% are funded by the BMDW through the Christian Doppler Research Association.
Selected Talks
- 23.02.2022: "Scientific Machine Learning" (online talk). Iași AI Community Meetup, Romania. DI Dr. Bogdan Burlacu. Slides
- 17.02.2022: "Welchen Beitrag kann symbolische Regression zu Explainable AI leisten? – Praxis-Beispiele aus dem Josef Ressel Zentrum" (online talk). Softwarepark Hagenberg IT-Expert*innenreihe „Thinking AI“, FH-Prof. DI Dr. Gabriel Kronberger. Slides
- 12.12.2020: "Genetic Programming and Symbolic Regression". AI Meetup Graz, FH-Prof. DI Dr. Gabriel Kronberger. Slides
Publications
2022:
C. Haider, F.O. de Franca, B. Burlacu, G. Kronberger. „Shape-constrained multi-objective genetic programming for symbolic regression". Applied Soft Computing, Volume 132, 2023, 109855, ISSN 1568-4946, https://doi.org/10.1016/j.asoc.2022.109855.
G. Kronberger, E. Kabliman, J. Kronsteiner, M. Kommenda. „Extending a physics-based constitutive model using genetic programming". Applications in Engineering Science, Vol. 9, 100080 (2022). doi: https://doi.org/10.1016/j.apples.2021.100080
F. Bachinger. „Comparing Shape-Constrained Regression Algorithms for Data Validation." Proceedings of the 18th International Conference on Computer Aided Systems Theory - EUROCAST 2022, Las Palmas de Gran Canaria, Spain (2022). Slides Paper
C. Haider. „Shape-constrained Symbolic Regression with NSGA-III." Proceedings of the 18th International Conference on Computer Aided Systems Theory - EUROCAST 2022, Las Palmas de Gran Canaria, Spain (2022). Slides Paper
D. Jödicke, D. Parra, G. Kronberger, S.M. Winkler. „Identifying Differential Equations to predict Blood Glucose using Sparse Identification of Nonlinear Systems." Proceedings of the 18th International Conference on Computer Aided Systems Theory - EUROCAST 2022, Las Palmas de Gran Canaria, Spain (2022). Slides Paper
L. Kammerer, G. Kronberger, M. Kommenda. „Symbolic Regression with Fast Function Extraction and Nonlinear Least Squares Optimization." Proceedings of the 18th International Conference on Computer Aided Systems Theory - EUROCAST 2022, Las Palmas de Gran Canaria, Spain (2022). Slides Paper
2021:
C. Haider, F. O. de França, B. Burlacu, G. Kronberger. „Using Shape Constraints for Improving Symbolic Regression Models” (2021). arXiv preprint arXiv:2107.09458.
L. Kammerer, G. Kronberger, S. Winkler. „Empirical analysis of variance for genetic programming based symbolic regression”. In Proceedings of the Genetic and Evolutionary Computation Conference Companion (GECCO '21). Association for Computing Machinery, New York, NY, USA, pp. 251–252 (2021). doi: https://doi.org/10.1145/3449726.3459486 Slides
W. La Cava, P. Orzechowski, B. Burlacu, F. Olivetti de França, M. Virgolin, Y. Jin, M. Kommenda, J. H. Moore. “Contemporary symbolic regression methods and their relative performance” (2021). arXiv preprint arXiv:2107.14351.
W. Roland, C. Marschik, M. Kommenda, A. Haghofer, S. Dorl, S. Winkler. "Predicting the Non-Linear Conveying Behavior in Single-Screw Extrusion: A Comparison of Various Data-Based Modeling Approaches used with CFD Simulations". International Polymer Processing. Vol. 36, Issue 5, pp. 529-544 (2021). doi: https://doi.org/10.1515/ipp-2020-4094
L. Millán, G. Bokuchava, J.I. Hidalgo, et al. "Study of Microscopic Residual Stresses in an Extruded Aluminium Alloy Sample after Thermal Treatment". Journal of Surface Investigation: X-ray, Synchrotron and Neutron Techniques. 15, pp. 763–767 (2021). doi: https://doi.org/10.1134/S1027451021040145"
E. Kabliman, A. H. Kolody, J. Kronsteiner, M. Kommenda, G. Kronberger. "Application of symbolic regression for constitutive modeling of plastic deformation”. In Applications in Engineering Science, Volume 6, 100052, Elsevier. (June 2021). doi: https://doi.org/10.1016/j.apples.2021.100052
G. Kronberger, F. O. de Franca, B. Burlacu, C. Haider, M. Kommenda. “Shape-constrained Symbolic Regression – Improving Extrapolation with Prior Knowledge”. In Evolutionary Computation (2021). doi: https://doi.org/10.1162/evco_a_00294
L. Millán, G. Kronberger, J. I. Hidalgo, R. Fernández, O. Garnica, G. González-Doncel. "Estimation of Grain-Level Residual Stresses in a Quenched Cylindrical Sample of Aluminum Alloy AA5083 Using Genetic Programming". In Applications of Evolutionary Computation (Conference Proceedings EvoApplications 2021), Vol. 12694, pp. 421.436, (2021). doi: https://doi.org/10.1007/978-3-030-72699-7_27
F. Bachinger, G. Kronberger, M. Affenzeller. “Continuous improvement and adaptation of predictive models in smart manufacturing and model management". In IET Collaborative Intelligent Manufacturing, Vol. 3, Iss. 1, Special Issue: Selected Papers from Collaborative and Intelligent Manufacturing in Industry 4.0 (ISM @SMM 2019), pp. 48-63, (March 2021). doi: https://doi.org/10.1049/cim2.12009
2020:
G. Kronberger, F. Bachinger, M. Affenzeller. "Smart Manufacturing and Continuous Improvement and Adaptation of Predictive Models". In Procedia Manufacturing, Volume 42. (2020). Part of special issue: International Conference on Industry 4.0 and Smart Manufacturing (ISM 2019). PDF Slides
L. Kammerer, G. Kronberger, B. Burlacu, S. M. Winkler, M. Kommenda, M. Affenzeller. "Symbolic Regression by Exhaustive Search: Reducing the Search Space Using Syntactical Constraints and Efficient Semantic Structure Deduplication". In Genetic Programming Theory and Practice XVII., pp. 79-99. Springer. (2020). PDF Slides
F. Bachinger, G. Kronberger. "Concept for a Technical Infrastructure for Management of Predictive Models in Industrial Applications". In Computer Aided Systems Theory - EUROCAST 2019, pp. 263-270. Springer. (2020). PDF Slides
M. Affenzeller, B. Burlacu, V. Dorfer et al. "White Box vs. Black Box Modeling: On the Performance of Deep Learning, Random Forests, and Symbolic Regression in Solving Regression Problems". In Computer Aided Systems Theory - EUROCAST 2019, pp. 288-295. Springer. (2020). PDF
G. Kronberger, L. Kammerer, M. Kommenda. "Identification of Dynamical Systems Using Symbolic Regression". In Computer Aided Systems Theory - EUROCAST 2019, pp. 370-377. Springer. (2020). PDF Slides
B. Burlacu, L. Kammerer, M. Affenzeller, G. Kronberger. "Hash-Based Tree Similarity and Simplification in Genetic Programming for Symbolic Regression". In Computer Aided Systems Theory - EUROCAST 2019, pp. 361-369. Springer. (2020). PDF Slides
L. Kammerer, G. Kronberger, M. Kommenda. "Data Aggregation for Reducing Training Data in Symbolic Regression". In Computer Aided Systems Theory - EUROCAST 2019, pp. 378-386. Springer. (2020). PDF Slides
J. Zenisek, G. Kronberger, J. Wolfartsberger, N. Wild, M. Affenzeller. "Concept Drift Detection with Variable Interaction Networks". In Computer Aided Systems Theory - EUROCAST 2019, pp. 296-303. Springer. (2020). PDF Slides
G. Kronberger, J. M. Colmenar, S. M. Winkler, J. I. Hidalgo. "Multilayer analysis of population diversity in grammatical evolution for symbolic regression". In Soft Computing . Springer. (2020). PDF
B. Burlacu, G. Kronberger, M. Kommenda. "Operon C++: an efficient genetic programming framework for symbolic regression”. In Proceedings of the 2020 Genetic and Evolutionary Computation Conference Companion (GECCO ’20), pp. 1562–1570, (July 2020). PDF Slides
2019:
W. Roland, M. Kommenda, C. Marschik, J. Miethlinger. "Extended Regression Models for Predicting the Pumping Capability and Viscous Dissipation of Two-Dimensional Flows in Single-Screw Extrusion". In Polymers. (February 2019). PDF
G. Kronberger, L. Kammerer, B. Burlacu, S. M. Winkler, M. Kommenda, M. Affenzeller. "Cluster Analysis of a Symbolic Regression Search Space". In Genetic Programming Theory and Practice XVI, pp. 85-102. Springer. (2019). PDF Slides
E. Kabliman, A.H. Kolody, M. Kommenda, G. Kronberger. “Prediction of Stress-Strain Curves for Aluminium Alloys using Symbolic Regression”. In Proceedings of the 22nd International Conference on Material Forming (ESAFORM 2019), Vitoria-Gasteiz, Spain. AIP Conference Proceedings. (2019). PDF
B. Burlacu, M. Affenzeller, G. Kronberger, M. Kommenda. “Online Diversity Control in Symbolic Regression via a Fast Hash-based Tree Similarity Measure”. In Conference Proceedings of the IEEE Congress on Evolutionary Computation (CEC), Wellington, New Zealand. (2019). PDF Slides
B. Burlacu, M. Kommenda, G. Kronberger, M. Affenzeller. “Parsimony Measures in Multi-objective Genetic Programming for Symbolic Regression”. In Proceedings of the Genetic and Evolutionary Computation Conference 2019 (GECCO ’19). ACM, New York, NY, USA. (2019). PDF Poster
G. Kronberger, L. Kammerer, M. Kommenda, B. Burlacu. “Visualisierung eines Suchraums für Symbolische Regression”. In Tagungsband Forschungsforum der Österreichischen Fachhochschulen (FFH 2019), Wiener Neustadt. (2019) Slides
S. Prieschl, D. Girardi, G. Kronberger. “Using Ontologies to Express Prior Knowledge for Genetic Programming”. In Proceedings of Cross Domain Conference for Machine Learning and Knowledge Extraction (CD-MAKE) co-located with ARES 2019, Canterbury, UK, August 26-29, LNCS. (2019). PDF
M. Kommenda, B. Burlacu, G. Kronberger, et al. "Parameter identification for symbolic regression using nonlinear least squares". In Genetic Programming and Evolvable Machines. Springer. (2019). PDF
G. Kronberger, S. Scheidel, C. Haider, M. Kommenda, M. Kordon. "Integration of Physical Knowledge in Empirical Models - A New Approach to Regression Analysis". In Proceedings of the 8th International Symposium on Development Methodology, Wiesbaden, Germany pp. 1 - 9. (2019) Slides
F. Bachinger, G. Kronberger. "Management von lernfähigen Vorhersage-Modellen für Industrie-Anwendungen". In Proceedings of The Future of Work, Education and Living, Linz, pp. 253-59. (2019).
2018:
B. Burlacu, M. Affenzeller. "Schema-based diversification in genetic programming". In Proceedings of the Genetic and Evolutionary Computation Conference, pp. 1111-1118. ACM. (July 2018). PDF Slides
G. Kronberger, M. Kommenda, A. Promberger, F. Nickel. "Predicting friction system performance with symbolic regression and genetic programming with factor variables". In Proceedings of the Genetic and Evolutionary Computation Conference, pp. 1278-1285. ACM. (July 2018). PDF Slides
F. Bachinger, J. Zenisek, L. Kammerer, M. Stimpfl, G. Kronberger. "Performance of industrial sensor data persistence in data vault". In Proceedings of the EMSS Conference, pp. 226-233. (September 2018). PDF Slides
Research
Symbolic regression is a data-based modelling method where the goal is to find a formula that describes given data. Similarly to other regression methods, the model allows to predict one or multiple variables given known values of the input variables. However, in symbolic regression one does not merely fit parameters to a fixed model structure. Instead, the goal is to identify the necessary model structure as well as optimal model parameters for the given dataset.
Symbolic regression means to find a simple symbolic expression (formula) that fits a given dataset. It is a supervised learning technique.
The term symbolic regression was coined by John Koza in the context of genetic programming. In later developments, different algorithm variants for symbolic regression have been proposed, many of which are based on evolutionary algorithms.
When using genetic programming, the user specifies which operators and basic functions are allowed to be used in the model. The algorithm starts with a set of random expressions. Through selection and random recombination the algorithm evolves a well-fitting model.
The resulting symbolic regression model might look like the following:
A symbolic regression model produced with genetic programming.
A drawback of evolutionary algorithms is that they are non-deterministic. For industrial applications we need deterministic and efficient parameter-less solvers for symbolic regression problems. In the Josef Ressel Centre we develop and implement such algorithms.
We take up the idea of “Prioritized Grammar Enumeration” (Worm and Chiu, 2013) which uses dynamic programming to create symbolic regression models. The algorithm uses a formal grammar as input which describes the structure of the symbolic regression models and is then able to produce all models for this structure up to a certain maximum size. The approach has potentially exponential asymptotic runtime for increasing formula sizes or number of variables. Therefore, heuristics are necessary to guide the search process to potentially more interesting parts of the search tree.
Prioritized grammar enumeration is a non-evolutionary solution methods for symbolic regression. Formulas are generated from a formal grammar, identical functions are detected via dynamic programming.
Applications
Symbolic regression is a general technique that can be used for modelling technical systems. In the Josef Ressel Centre we use it for modelling components of powertrains.
An example for a hybrid power train with engine, electric motor with battery, transmission and differential drive. Modern powertrains are made up from many complex components and there are many possible design variants. Models are necessary for simulation of powertrains in a virtual design process. We use symbolic regression e.g. for modelling engine performance, friction performance, or battery load state.
For this we use data from testing benches as operated by our company partners AVL and Miba.
Testing bench for friction plates as operated by Miba Frictec in Roitham. Foto: © Bernhard Plank (http://imBilde.at)
Three company partners participate in the proposed JRC. AVL List GmbH (AVL) is the largest independent company for development, simulation and testing technology of powertrains. AVL uses various methods for regression modelling for powertrain development. We investigate whether symbolic regression models can be implemented successfully on engine or transmission control units for optimized control of powertrain components.
Miba Frictec GmbH (Miba) produces friction materials and components for friction systems as used for instance in clutch and automatic transmission systems, and provides services for the design and dimensioning of friction systems. Miba integrates the results of the JRC into their in‐house software‐tools so that Miba engineers are able to easily create and validate symbolic regression models for friction systems.
EREMA Engineering Recycling Maschinen und Anlagen GmbH produces and develops systems and components for the recycling industry. Based on large amounts of data from EREMA plants in the field, they develop software and methods for automated evaluation, interpretation and modeling.
HeuristicLab
The methods developed in this project are intergated into the open-source software framework HeuristicLab.
HeuristicLab download statistics (March 2018)
Further Information
Project description on the CDG website:
https://www.cdg.ac.at/forschungseinheiten/labor/symbolische-regression/